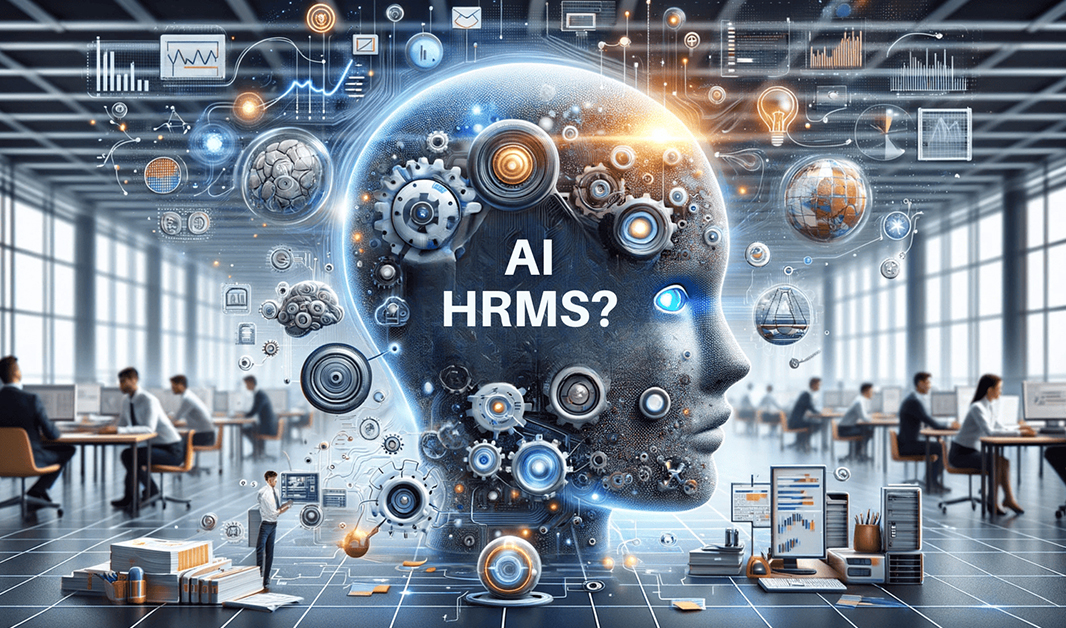
Can AI Truly Eliminate Hiring Bias?
AI significantly reduces hiring bias through unbiased data-driven assessments, but fully eliminating bias also requires human accountability
As more businesses worldwide adopt AI-driven hiring tools, conversations around bias within recruitment processes have intensified. Hiring bias—whether implicit or explicit—is a persistent concern that affects organizations globally. The presence of discrimination in recruitment can restrict workplace diversity, innovation, and even profitability. This reality has generated rising hope in using artificial intelligence (AI) as a neutral way to screen, analyze, and select job candidates, but can AI genuinely eliminate hiring bias once and for all?
Unconscious biases inevitably infiltrate traditional hiring practices. Human recruiters, regardless of expertise and intention, are prone to subtle psychological preferences, stereotypes, or subjective evaluations. A candidate may unintentionally be favored due to shared educational backgrounds, similar interests, or merely a pleasant personality. Yet such hidden biases can severely limit the diversity and quality of hires. This is where AI has promised to come into the picture, advocating a solution fueled by impartiality supported by data-driven methods. However, before confirming AI technology as completely bias-free, we must evaluate it carefully in the context of today's business realities.
It's true that AI hiring tools offer distinct advantages over manual recruitment methods. For instance, an appropriately designed AI hiring pipeline evaluates candidates objectively against preset role-specific criteria, basing decisions exclusively on verified experiences, competencies, and measurable skills rather than subjective feelings. Such practical clarity minimizes unintentional favoritism, enabling organizations to adopt a transparent and standardized selection method and helping foster workplaces that reflect diversity in talent.
Yet, AI is not inherently bias-free. It's important to understand that AI is developed, programmed, and trained by humans—and that direct human involvement indirectly means certain biases may inadvertently enter into the algorithm through the datasets chosen for learning or the underlying assumptions in the programming itself. AI models depend heavily on large historical datasets to train their decision frameworks. If such datasets possess historical biases—say, previous hiring trends that favored a particular gender, ethnicity, or education background—that bias becomes inadvertently embedded within the AI-driven recruitment approach. Without careful checks and balances, these biases replicate themselves, creating perpetuated discriminatory practices rather than addressing them. Recognizing this risk is crucial, for the trust and acceptance in AI recruitment hinges directly on ensuring fairness at every stage.
Fortunately, technology advancements and ethical AI developers acknowledge this problem and have increasingly begun to tackle bias-related issues proactively, emphasizing fairness and transparency. To minimize unconscious biases, AI developers have enhanced algorithms to detect disparities or imbalances within existing datasets and decisions. Techniques including fairness constraints, algorithmic audits, bias testing, continuous monitoring of outcomes, and dedicating expert oversight thoroughly evaluate hiring technologies before their deployment. Data scientists also perform repeated checks and balances by reviewing input sources, emphasizing fairness metrics, benchmarking against ethical standards, and meticulously assessing implemented recruitment technology for adverse impacts—a significant stride in combating bias proliferation.
At the same time, complete dependence solely on technology may not be wholly sufficient. AI-driven hires should be envisioned more as a team effort between human judgment and digital decision-making. By positioning recruiters alongside AI tools, organizations gain a unique balance of impartial data-driven insight and empathetic understanding. Humans are naturally equipped with emotional intelligence, nuanced insight, and ethical reasoning that AI currently lacks—a considerable factor when comparing potential hires with diverse backgrounds. Human resource experts’ judgment and sensitivity, together with AI-powered analytical rigor, create strengthened employee selection processes vastly more robust than either method working independently.
The role of AI should thus be reframed not as eliminating human involvement in hiring but instead empowering recruiters to address hidden biases knowledgeable and thoughtfully. AI systems flag potential biases, identify overlooked qualified candidates, and offer vital analytics on recruiting trends—informing human recruiters and hiring managers of potential blind spots existing within current practices. Adjustments can then be made proactively. Organizations cultivating such collaborative relationships between technology and humans build hiring processes defined by transparency, fairness, integrity, and inclusivity.
In the broader scope, organizations ought to integrate regular training about unconscious biases within their recruitment and talent acquisition teams. Educating people around these deeply-rooted inherent biases, organ¬i¬za¬tion¬al values of inclusive workplace culture, and AI ethics enables recruiters, alongside AI platforms, to cooperatively curb the spread of discriminatory hiring behaviors. Only through dedicated human commitment combined with ethical design and transparent deployment of AI systems can hiring committees successfully achieve meaningful diversity initiatives.
Conclusively, it becomes apparent that AI alone is not the entire answer to eliminating hiring bias—it's a significant part of the strategy but must be balanced by credible human oversight and constant quality revisions. AI, when suitably trained, ethically designed, transparently audited, and thoughtfully applied, becomes an extremely powerful tool for drastically minimizing biases within recruitment processes. A holistic approach—founded upon enriching technology, reinforcing human accountability, continual awareness, and communication—remains the most realistic and effective approach to combating biases, ultimately cultivating workplaces driven by fairness, equity, and growth.